What It Means When We Talk About Student Data
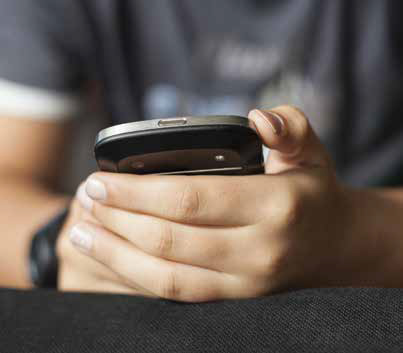
Within educational technology, tech companies can acquire data from multiple routes. The most direct way is via a direct signup. A teacher creates an account to use a service, and the teacher is the only person using the service. BetterLesson is an example of a site like this. A teacher creates an account, and only that teacher’s data is collected. The site is primarily teacher-focused.
Web sites also pull their initial data from teacher or school signups, but then as part of the service offered on the site, they acquire student information. Basic gradebook applications and some simple student information systems work like this. The teacher or school signs up for the application, and in the process of using it, they enter student names, grades, notes, parent information, and other details. The actual data added will vary based on the needs of the application, but information is shared about people without their direct involvement or consent. Online IEP programs also fit this description. While the teacher or school provides the original input for the account, the vendor ends up getting additional data through the teacher’s use of the account. In this model, students and parents do not use the service directly, but data about them is collected and stored in the account as teachers use the service.
Data can also be collected when a teacher, school, or district creates an account on the service, and either creates accounts for their students and parents or uses an invitation process. In this version, teachers, students, and potentially, parents sign up for and interact with the service. The data trail here involves information about all participants that includes personally identifiable information, location data (via IP addresses and/or phone GPS location), and behavioral and interaction data pulled from time spent using the service. Examples of services like this include many edtech products, including Edmodo, Remind, ClassDojo, Schoology, most digital text offerings from traditional publishers like Pearson and McGraw Hill, learning programs like Agilix Buzz, and app ecosystems like Amplify tablets, iPads, and Chromebooks.
Learner and parent data can also be compiled from apps for kids that are marketed either to parents or to children. Examples of applications like this include most educational apps sold in the Apple and Google app stores, and some online learning sites. Because these apps are built to be used outside of schools, the data collected by them is not considered an educational record under FERPA. However, there are safeguards in place that are covered by the privacy policies and terms of service provided by the app vendor. If the app is primarily intended for children under 13, parental signoff (and therefore parental data) is often required for use.
Highly structured datastores to collect longitudinal data, and glue services that integrate multiple external services, are other ways that personal data can be harvested. These applications can support both storage of and analysis on data collected in a variety of applications. A partial list of examples includes Knewton, Infinite Campus, eScholar, Schoolnet, Learnsprout, and Clever.
THE CONTEXT OF EDTECH DATA COLLECTION
The context around educational data is arguably different than for data collected in consumer technology. In both K-12 and higher ed, schools can sign up for services that students use directly, and in many cases, student data is uploaded before students or parents are consulted. For example, if a teacher signs up for Remind, parents aren’t asked if their contact information can be shared as part of an “invite” feature. While many consumer tech apps include invite features, edtech apps are used within a different context. When a student or a parent sees an app or an invite coming from a school or a teacher, there is a level of implied trust. Increasingly, the implicit trust that students and parents give schools and districts appears to be unearned.
DATA PRIVACY PLAYS OUT OVER TIME
Unless data collected by an app is deleted or destroyed, and this includes data in backups and in systems that provide redundancy, we need to start thinking of data trails as timeless. This means that a data trail can be transferred from one entity to another if the conditions allow these transfers to occur. In technology, the terms and conditions and privacy policies are where we can see the ways in which our data trails are preserved.
While privacy policies and terms of service should be read in full (such as for free logins that are required for access), for the purposes of this discussion we are going to focus on two specific sections that can be used to gut the terms in any policy— how policies can be changed and how data is treated in case of sale, merger, or bankruptcy.
CHANGES TO TERMS
Over time, a site’s policies may change. Unfortunately, many sites specify that terms can be changed at any point, with no notice to end users and with no explicit signoff from end users. Many sites state that visiting a site or logging into a site means that a user accepts the updated terms. The simple act of reading updated terms of service is now interpreted as “acceptance” of the terms. Even on sites that have better notification policies (and at this point, the “best” policies generally include an email and a banner on the top of the site), users often have no recourse (aside from stopping their use of the site) if they don’t like the updated policies. Additionally, many sites do not allow users to delete their data from a site, so even if they stop using a site, their data is still stuck in the site.
The following example from ShareMyLesson Privacy Policy uses pretty typical language:
From time to time we may be required to, or need to, update this Privacy Policy. Your continued use of the Service after we post a revised Privacy Policy signifies your acceptance of the revised Privacy Policy. It is therefore important that you review this Privacy Policy regularly to ensure that you are aware of any changes. If we materially change our practices regarding collection, use or disclosure of your personal information, your personal information will continue to be governed by the Privacy Policy under which it was collected unless you have been provided notice of, and have not objected to, the change. Where necessary and appropriate we may contact you through the email address that you have provided, to advise you of a change. All changes will be accessible from the Service through the Privacy Policy and notification of the last date of change placed at the top.
Most sites reserve the right to change their terms of service whenever they want, with minimal notification, and no option to remove data. In the case of a site where a learner has been added to a site by a school or district, learners have even less recourse.
DATA TRANSFER DURING SALE, MERGER, OR BANKRUPTCY
While the sale, merger, or bankruptcy of a company are three very different events, most privacy policies treat them identically. If these conditions happen, user information is an asset that gets sold.
The following privacy policy from Edmodo is pretty standard for edtech terms:
5. Business Transfers
If Edmodo, or some or all of its assets were acquired or otherwise transferred, or in the unlikely event that Edmodo goes out of business or enters bankruptcy, user information may be transferred to or acquired from a third party.
To start, the weak terms used throughout edtech could be improved by implementing the following four changes:
■ Users can opt in to changed terms and/or export data as part of an account cancellation process.
■ Users opt in when data is transferred to a new owner.
Users can access account cancellation or data deletion as a regular feature within an app.
■ In the case of a bankruptcy or the sale of a business, the user’s data is destroyed and not treated as an asset.
These four changes would ensure that user awareness and buy-in are included as factors when terms are changed. There are additional ways that vendor practices could be improved, but starting with these four recommendations would be a solid beginning.
When a student is signed up for a service by a school or teacher, data is collected from people who have no say in forming the relationship or shaping the terms of the deal. In some cases, this involves student work being sold without student knowledge. The fact that edtech companies treat student data (which really is a track record of learning, personal interests, and growth) as an asset to be bought or sold is on very shaky ground, both pedagogically and ethically. Given that a learning record is also a snapshot of behavior, and that behavioral info is gold for marketers, it raises the real question—why should education records ever have the possibility of ending up outside an educational context? Treating records as a financial asset that can be acquired in a merger or bankruptcy ensures that some records end up being used outside an educational context. The combination of the “fail faster” mantra of VC-funded tech, and the ongoing deals reaching over 8 billion dollars worth of revenue in 2014 alone, guarantees that student data is getting sold and used outside an educational context.
When a technology company reserves the right to sell user data in case of bankruptcy, they are hedging their bets. By claiming a stake in user data, instead of getting firmly behind their product or service, they are telling us that they do not have complete confidence in their product. When a company tells us that, we should listen and return the favor. If a company doesn’t have enough faith in their product to leave user data off the table as an asset, we should match their level of faith and not use their product.
WHO CARES WHERE DATA ENDS UP? IT'S ONLY SCHOOL!
Data Brokers Care. They have created lists of victims of sexual assault and lists of people with sexually transmitted diseases. There are lists of people who have Alzheimer’s, dementia, and AIDS. You can find lists of people who are impotent and lists of the depressed. There are lists of “impulse buyers.” You can search for lists of suckers, also known as gullible consumers, who have shown that they are susceptible to “vulnerability-based marketing.” There are even lists of those who are deemed commercially undesirable because they live in or near trailer parks or nursing homes. Not to mention lists of people who have been accused of wrongdoing, even if they were not charged or convicted.
In the housing market, we have examples where information from data brokers was used to discriminate loan approval based on race. If you’re looking for a relatively benign example (if you can get through the marketing speak) of how data from brokers can be mashed up to create profiles, spend some time on this zip code profiler put out by ESRI. It’s worth noting that the profiles were created based on aggregated data of individuals, so that the summaries here are the result of millions of data profiles on individuals. The description of how the site was put together provides a superficial glimpse of how data on individuals from multiple sources can be combined to tell a story.
Now imagine the increased accuracy that could be added to personal profiles if they were fleshed out with datasets that contain personal information, starting with habits formed in elementary school.
The life of a data trail matters.
Tools and ideas to transform education. Sign up below.